From Data Lake to Data Mesh
Reading Time: 14 Minutes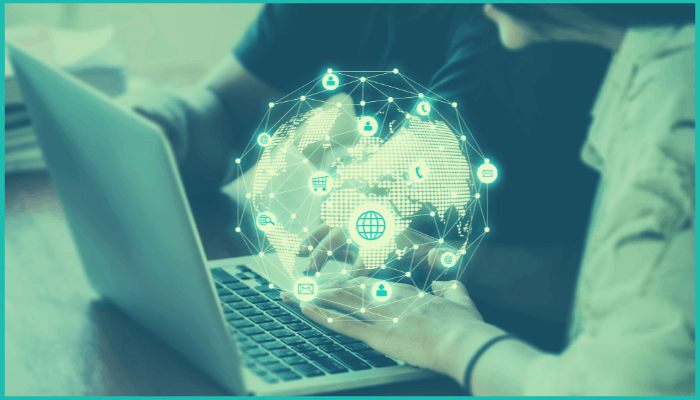
At Bungee Tech, we collect, cleanse, enrich, and index retail data using our patented data collection platform.
Our state-of-the-art ML system creates a retail market map, provides rich insights, and performs autonomous actions. These are exposed using our well-architected SaaS platforms (embracing 6 pillars of architecture and clean code architecture).
We host a wide array of key datasets in the retail vertical:
- Analytics/actions for global pricing and promotion
- Global product catalog and assortment
- Global brand catalog
- Global category catalog
- Inventory and availability
These retail domain datasets are rich in business value and are truly big data in nature.
What is a data lake?
A data lake is a centralized, domain-agnostic data persistence architecture that allows you to store structured and unstructured data at scale. It separates storage, and computes to scale for huge volume, and accommodates varied load and access patterns – all at a reduced cost.
What is data mesh?
Data mesh is an industry-leading approach to data management. It defines a clear domain-based design paradigm to group and manage datasets ownership. Data mesh treats datasets like product – all powered by a self-serve data platform and governed by a federated governance mechanism to effectively scale the data operations of an analytics organization.
Our Data Journey: The Beginning
We started by hosting datasets in a data lake, which provided immediate benefits:
» Flexibility – hosting structured, unstructured, and/or semi-structured datasets in a centralized lake.
» Viability – separating storage and compute to accommodate different usage and load patterns across the organization.
» Availability – executing as a fast-paced startup with incredible cost benefits compared to the previous generation enterprise data warehouse architecture, solutions, and tools.
We started with a highly decentralized execution model that helped us move fast and rollout tons of advanced capabilities in a short period of time.
But it came with a few problems: data duplication, source proliferation, data quality and integrity divergence between related sources and a bunch of domain agnostic data ownerships. We quickly identified these issues and consciously created focus groups that followed a loose domain ownership model. The split of these focus groups were based on the “data pipeline architecture/organization model”.
As highlighted in the data mesh paper, the above pipeline architecture/org structure might appear to be an effective ownership model initially. However, in practice, all the focus groups must work to launch even very small, new functionality. This created a siloed hyper-specialized data platform team with very little understanding of the source domains that generate the data. They lack the domain expertise of the analytics consumption teams that they cater analytics to. This limited our ability to achieve our ideal speed and scale.
Data lakes are no longer the centerpiece of the overall architecture of a matured analytics ecosystem. The data lake architecture fails to gracefully accommodate changes in the data landscape and leads to proliferation of sources of datasets within the organization and impedes the speed of response to change.
The Present & The Future
To provide a truly decentralized architecture that avoids the above mentioned issues, we came to a conclusion that data mesh is the right data architectural and organizational pattern. Data mesh fits Bungee Tech’s needs in the short and long term.
“At Bungee Tech, we’re happy to have business and tech alignment in our core operating model. We have a data-oriented strategy where we are convinced beyond doubt that quality data, ML, and advanced analytics form our strategic differentiator in the market, explains the company’s CTO, Venkat PK. He continues that the company’s executives are “spearheading data maturity models within the organization and have a long-term commitment to invest in advanced architectural/organization transformations like data mesh in the right form and shape.”
4 Pillars of Data Mesh @ Bungee Tech
1. Domain – Domain oriented data decomposition and ownership
The entire data ecosystem is grouped and tagged to source-oriented domain data, consumer-oriented domain data, or shared domain data. In the process, we have domain-based data ownerships. There are clear rules on who should own any new dataset requirements in the organization. This process stops any inefficient data set proliferation in the organization.
2. Data as a Product – Data and product thinking convergence
Data is given due respect, wherein data is treated as a product. It is assigned to the proper domain using a clear rule and is properly addressable and discoverable. Its structure, both logical and physical, must be defined with utmost discipline. Lineage of data and transformation rules must be defined and maintained. Quality rules, thresholds on breach of these rules, and related alarms become first-hand citizens to make the data truthful and trustworthy. E2E operational aspects like any changes in statistical shape of data, observability, freshness, retention, dev ops are key aspects to be mandatorily defined and structured for every data product. Security of the data with proper classification and related treatment such as encryption, global access control is mandated.
With this, the key focus in Bungee Tech shifts to the data within a domain. The pipelines become the data product’s internal implementation.
3. Data Platform – Data and self-serve platform design convergence
At a physical layer, data mesh’s self-serve data platform provides access to scalable polyglot data storage, data products schema, data pipeline declaration and orchestration, data products lineage, compute and data locality, etc.
At a logical level, there are proposals in the data mesh paper to have a multi-plane architecture that includes layers like data infrastructure provisioning plane, data product developer experience plane and data mesh supervision plane to name a few.
At Bungee Tech, we use our existing cloud service capabilities to drive the platform aspect of this transformation for now. But, in the months to come, based on our experience in the transformation process, we are motivated to make the right investments at a platform level to facilitate this transformation without any friction.
4. Federated computational governance – Make decentralization work efficiently
Data mesh completely decentralizes the governance aspect of the data as a product. It relies on federated custodian of data governance by domain owners. The domain owners define how to model data quality, data security/monitoring, model polysemes, reliability, and operational excellence of data as a product.
Despite such localized decision making and autonomy, they need to comply with the standard defined by the global federated governance team and automated by the platform.
Bungee Tech has created domain-based ownership and key point of contacts in each of these domains to put together a global federated governance.
We’ll keep maturing and transforming these pillars.
The Data Mesh Pitfall to Address
Data mesh tries to address most of the pitfalls associated with decentralized architectures via the power of a matured data platform. But, building and embracing such platform capabilities can take some time. The challenge in decentralizing specialized roles (data engineers, data scientists, etc.) based on domains in an organization limits communication and coordination in specialized job families.
It reduces opportunities for collaborative learning and structuring a proper growth path for these specialized roles. This could eventually lead to poor data standards and reduce the pace of execution of data related problems without organizational maturity. Bungee Tech is cognizant of these key issues with data mesh when it’s not backed by a full-fledged data platform and is working on an operating model to address this.
References:
The Latest Insights – Straight to Your Inbox
Sign up for the Bungee Tech mailing list for actionable strategies, upcoming events, industry trends, and company news.