Harnessing Generative AI and Machine Learning for RGM Success
Tech-backed revenue growth: a favorite topic of ours.
Sure, machine learning and generative AI are buzzworthy terms, but they’re also powerful applications used by retail businesses and CPG manufacturers to drive revenue growth.
Since ChatGPT went live last November, this whole area of technology has been the “it” topic.
Many of us understand the core concept, but what’s apparent is that we’re still wrangling with how to take advantage of this technology to get to the next level.
Retailers & manufacturers share a common goal: staying competitive in the market and boosting profitability. AI and machine learning tools will help you do just that.
Rather watch than read?
🎥 Watch on YouTube: Harnessing Generative AI & ML for RGM Success webinar here
Evolution of AI
So While the concept of RGM has been around for years, generative AI and machine learning are newer.
By 2030, the generative AI market alone is projected to reach more than $110 billion dollars. Experts are also saying that in a couple of years, Generative AI will account for 10% of all data produced, that’s 10 times more than just a few years ago.
“AI is already transforming the retail market behind the scenes with traditional AI/ML improvements. Generative AI simply adds rocket fuel to that potential financial impact,” says Greg Buzek, president of IHL Group.
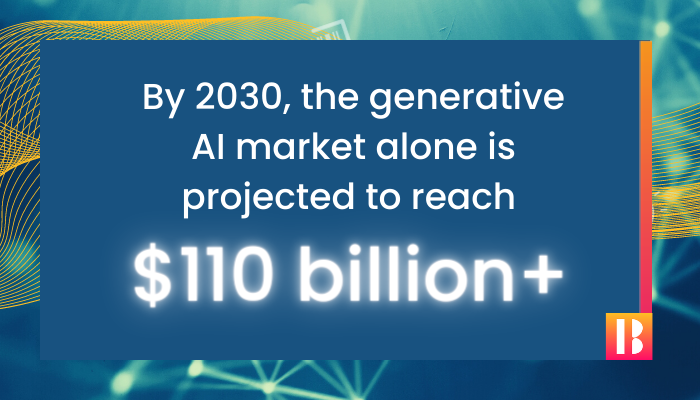
All the hype around this tech is warranted because a report by BCG and Google indicated that companies that deploy AI and ML applications at scale could boost revenue by at least 10%.
Drive Retail Results
Regardless of technology, there’s a few core truths that hold true for shoppers…
They want a seamless experience.
To win the moment of purchase and elevate above the competition you need to focus on…
- Speed & convenience: It’s easy and quick to shop the store. There’s an efficient checkout process. The actual location is convenient.
- Assortment & selection: Great product selection/quality.
- A Digital influence: Awesome app experience. Great shipping terms.
- Operations: Consistency in brand / product availability. Consistency in pricing across channels.
- Cost: Price, promotions, and rewards play a role to win the shoppers dollars.
Let’s zero in on that cost influence…
Price-Value
Many consumers say that price is their biggest consideration when they’re choosing where to shop and what to buy. I’m sure you have been either involved in many conversations in your own work on the price and cost of goods, especially in the last 18 months. And this affects everyone here – I mean, it wasn’t that long ago that we all freaked out at the price of eggs!?
Cost pressures related to inflation are a big reason price is so important to your consumers.
A recent McKinsey study showed that consumer confidence is temperate at best, but they are also spending less across the board.
And while recently we learned that inflation only rose 0.2% in June, it hasn’t stopped shoppers from caring about price. But it’s more than just price – what most customers really regard is VALUE.
That means that shoppers might spend the same or more on products if they realize the offer gives them value for the spend.
Whether a retailer or manufacturer, your mandate therefore is to strategize around that so that you’re able to win the moment of purchase – and ultimately create that growth you are looking for.
How Can ML and AI Help?
Clear business use cases are emerging for applying machine learning in each area of retail operations.
Especially for merchandising, a dominant signal into RGM.
According to Grocery Doppio, the top use cases of ML / AI are:
- Pricing & promotion optimization
- Assortment optimization
- Planogram optimization
Deep Learning Models
Machine learning refers to deep learning models.
Deep learning uses AI neural networks are inspired by the human brain. These models learn to perform tasks and make predictions by learning from data. They can handle more complex patterns compared to traditional models.
Can be trained on small amount of labelled data and large amount of unlabeled data and uses a combination of supervised, unsupervised and semi-supervised techniques.
There’s 2 types of deep learning…
-Discriminative
-Generative
One model, discriminative, does tasks like classification & prediction. For example, discriminative AI will look at something and say if it’s an apple or not.
Then the other model, generative AI, will basically say I will generate an apple based on what’s been learned in the past.
We need both models for long-term retail operations.
As a real world example, lets talk about assortment gap management.
You need to take your catalog and then match it to the rest of the market to find the assortment overlap and gap with your competitors.
You need discriminative models to do product matching that is necessary for generating this overlap and gap set.
Once you onboard an assortment gap and want to create a listing page for it you need a generative model.
Another example is that you may decide to run a promotion looking at your competitors offer and that is a discriminative model output.
Creating fabulous product page content for the promotion is a generative model output.
These models go hand-in-hand. The combination of both is where revenue growth begins.
Your RGM Strategy
Typically, revenue growth management focuses on key operational elements like price, trade spend, and assortment. Managing these elements helps you better understand success drivers, identify new – profitable – opportunities.
“RGM isn’t a new concept, it’s been talked about for many many years. It’s not adopted widely because of cost. Acquiring competitive data can be expensive. Then it’s the process of ingesting that data and the need of people who can manage and plan with that data. So, if you think about your own trade planning… you’re probably only doing one, two, trade planning sessions a year (at best). That’s because it takes a long time – to create elasticity models, to see the competitive landscape, to understand trade budget with finance, and then actually planning the events and getting it approved. So – the generative AI / machine learning side is the accelerator for me. The winners are going to adopt tech-enabled data to drive revenue growth.”
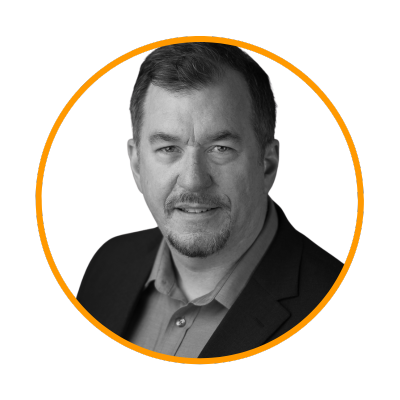
And if you relate the price/value conversation – it’s important not to lose sight that one of your RGM-based goals : provide that unmatched price-value perception for your targeted customer that maximizes for profit and margin while continuing to drive a purchase decision, create a connection with your shopper that inspires loyalty and repeat business.
So there’s some real opportunity for revenue growth / category management / pricing leaders to optimize performance with data.
Machine Learning & AI via Competitive Intelligence
The real differentiation for companies driving growth vs. those struggling to generate positive outcomes starts with high-quality data.
In the past that has been a high cost to acquire due to the complexities around collection, processing and then delivering that data. E-commerce made that 10 times harder where retailers now have their own classifications and product hierarchies that differ. Who here wants to match every UPC in their competitive set to an ASIN in Amazon? It’s a massive task and changes every single day.
The secret weapon is being able to trust & leverage quality data collection, product matching, and then the output of actionable analytics through an adaptable process that connects to your business and scales effectively so that you are not burdened with the operational costs.
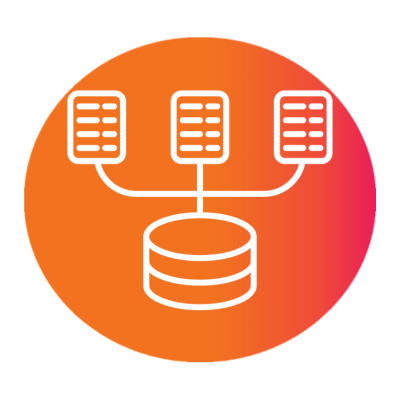
Reliable, Uninterrupted
Data Collection
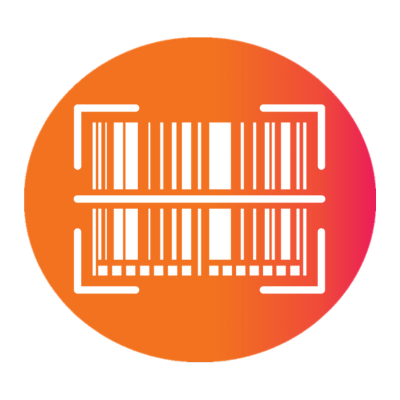
Accurate Product Matching Capabilities
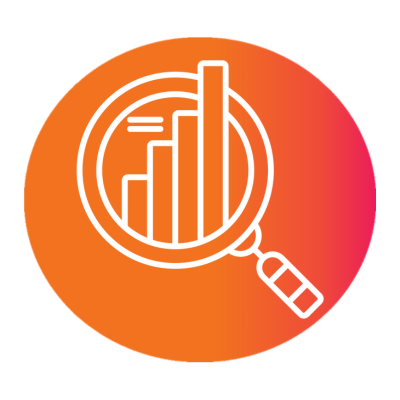
Actionable Analytics Driven by ML
Product matching is a foundational problem for many businesses. Despite tech advancements in catalog management, we see that general catalog quality is pretty poor. So many things can (and do) go wrong – missing titles/descriptions, the wrong UPCs, and the wrong categorization or prices. It’s challenging to manually reconcile these issues.
Your ability to compete in the market – without sacrificing margin or losses – is directly related to accurate product matching.
Whether you’re trying to match prices against your biggest competitor or you’re ready to onboard a great, new product to close the assortment gap… the product match process needs to be perfectly accurate.
That’s where we come into the picture…
Bungee Tech has built multimodal machine learning models which use both discriminative and generative AI capabilities. We’ve been at this for years… training our models on different retail data sets. You can match exact, equivalent, or similar products. You can have matches against national brands and private labels – any combination.
In addition to the machine learning models, it’s extremely important that we have a human feedback loop to audit the process. We’ve built necessary applications and workflows so category managers can review the output of the machine learning models. With feedback, the model keeps learning and becomes better over time.
Insights from the panel
“Think back to the start of Covid, when we pulled back on promotions. Now, promotions are ramping up as we look to see that consumers are pulling back on their spending. Everyone’s asking – how do we get more out of our system? But I challenge everyone to think about the reason why they want to promote. It should never be because we did it last year, so we’ll do it again. It shouldn’t be to keep space. Trade dollars are something to protect – something to use the right way – and something to manage. Taking a long time to create that promotional plan means dollars will leak away. The place we need to get to – in trade, in pricing – is allowing technology like AI to help us accelerate planning. That happens with the reliable data collection so we can make value-added decisions. At the end of the day, the challenge is to get out of the legacy stuff, and getting in with the program that will drive you forward.”
-Paul Morgan, Champion Petfoods, CPG analytics expert
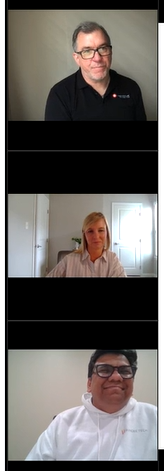
What Do You Do Now?
If you’re interested in a scalable, accurate data collection and product matching process – reach out to us today ([email protected]). We’d love to connect with you, learn your challenges, and find ways to use technology-enabled data to solve for them.